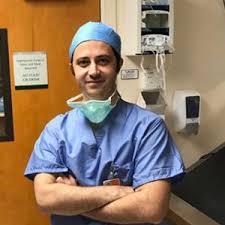
Eyüp Özcan MD
Despite the accuracy of Placido-disk based corneal topography and tomography imaging for diagnosing keratoconus, post-LASIK ectasia remains a challenge, occurring at least 0.033% (10/30,000) of cases. “Therefore, a better evaluation is required to detect keratoconus, especially in the early stages,” said Eyüp Özcan MD, at the ASCRS Virtual Annual Meeting 2020.
To that end, Dr Özcan and colleagues at the Bascom Palmer Eye Institute, University of Miami, Florida, USA, developed an artificial intelligence tool to autonomously assess corneal images for keratoconus signs. While autonomous systems exist for diagnosing diabetic retinopathy, glaucoma, age-related macular degeneration and retinopathy of prematurity, no autonomous system has been reported for keratoconus, he added.
Hidden features
Using techniques of deep learning based on analysis of thousands of training images, the AI tool identifies hidden features in the data set that may be missed by unaided human analysis, Dr Özcan said. His group built on existing criteria in anterior segment OCT imaging for detecting keratoconus features (
Sandali, O et al. Ophthalmology 2013; 120(12):2403-2412), and applied it to detect keratoconus autonomously using high-definition OCT images generated by a Bioptigen Envisu R2210.
Data from labelled training images were assessed by a deep neural network. The resulting algorithm, Bascom Palmer AI 1.0, was then applied to assess 7,740 images from 43 eyes of 23 patients with keratoconus and 5,040 images of 28 eyes in 28 patients without keratoconus. Results were compared with diagnoses provided by board-certified corneal specialists at Bascom Palmer.
While one eye of a keratoconus patient was diagnosed as healthy, the fellow eye of that patient was correctly identified with keratoconus.
“So, the algorithm was able to correctly differentiate all patients with keratoconus from healthy subjects and thus achieving accuracy, sensitivity and specificity of 100%,” Dr Özcan reported. Similarly, it correctly diagnosed 42 out of 43 keratoconic eyes, achieving accuracy of 97.9%, sensitivity of 95.3% and specificity of 94.6%.
More Data: Increased accuracy
Separately, Renato Ambrósio Jr. MD, PhD, Adjunct Professor of Ophthalmology of the Federal University of the State of Rio de Janeiro (UNIRIO), Brazil, reported on a machine learning approach to further optimise the current integrated Scheimpflug tomography and biomechanics index (TBI). The seminal TBI study included one eye randomly selected from 480 healthy patients, from 204 keratoconus cases, and 94 eyes with typical or normal topography from patients with very asymmetric ectasia (VAE-NT). To further enhance the accuracy of ectasia detection, a large cohort of cases from the International Corneal Tomography and Biomechanics Group included one eye randomly selected from 1,679 healthy patients, from 1,084 keratoconus cases and 543 VAE-NT cases. Expanding the data does allow for optimising the AI methods. This study was done with the BrAIN Med (Brazilian Artificial Intelligence Networking in Medicine; www.brain.med.br).
A random forest (RF) method based on 16 clinical features increased the sensitivity in detecting abnormality in normal-topography eyes in very asymmetrical ectasia cases from 75.7% to 85.6%, with a higher specificity of 90.5%, compared to 89.1%. For the clinical ectasia cases, the new RF also augmented sensitivity from 98.4% to 99% and specificity from 98.5% to 99.2%.
“The aim of the TBI is to provide an objective metric for epitomising the susceptibility for ectasia progression, which goes beyond but not over detecting keratoconus,” explained Prof Ambrósio.
“We have to be very careful with internal cross-validation methods when programming AI, which requires a large data set. Indeed, external validation tests are always to be considered, and further enhancements with continuously more massive training sets should be expected. Also, improvements should come with the integration of additional parameters including wavefront data, axial length, epithelial thickness data is part of the plan, along with the correlation with genetics and finite element analysis,” added Prof Ambrósio. “This is truly a revolution in evolution,” he concluded.