Artificial Intelligence
The Symphony of AI in Ophthalmology
Henahan Prize Finalist Essay
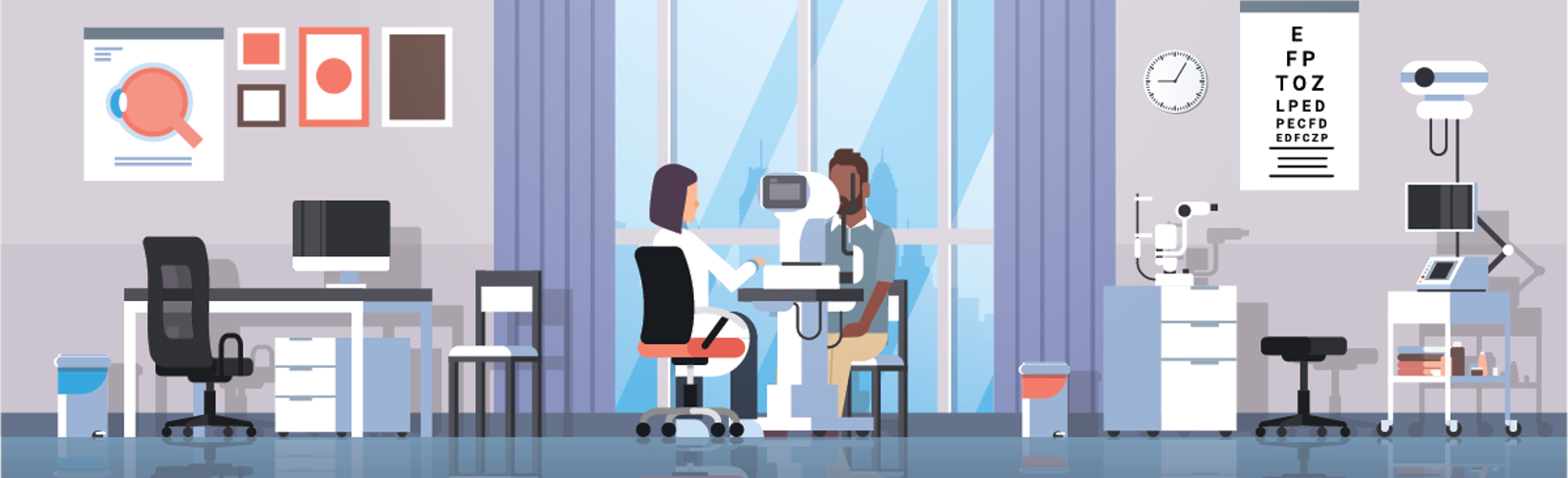
Dr Liu submitted this essay to the John Henahan Writing Prize essay contest, answering the prompt “What is the potential role for AI in ophthalmology, and what are the negative implications and caveats?”. It was rated in the top 5 of 41 essays submitted by the medical editorial board of EuroTimes.
By Siyin Liu MD
Medicine is at a critical inflection point for artificial intelligence (AI). With a whopping 3,327 new AI companies in the mix and a projected $37 billion splurge on AI by 2025, this tech is drastically transforming every industry, including healthcare.
Ophthalmology, with its rich imaging data, presents an ideal setting for training algorithms in image recognition, segmentation, and disease detection. Current focus lies on prevalent ophthalmic conditions like diabetic retinopathy (DR), age-related macular degeneration (AMD), and glaucoma, leveraging large, standardised imaging data sets. The COVID pandemic accelerated the integration of AI into tele-ophthalmology, exemplified by the FDA-approved autonomous diagnostic device for DR, enabling point-of-care diagnosis without human oversight. Challenges exist in AI research for anterior segment diseases due to non-uniform slit-lamp images and limited data sets. Nevertheless, recent advancements demonstrate AI’s potential in the anterior segment, including early detection of keratoconus, post-refractive surgery ectasia screening, and diagnosis of infectious keratitis.
AI in ophthalmology has primarily focused on image-based deep learning. Yet, the adoption of electronic health records has unveiled the untapped potential of unstructured free-text data. Natural Language Processing (NLP) and algorithmic rule-based text extraction techniques have shown promise in leveraging this data to enhance care delivery and for big data analysis, predictive modelling, cohort identification, and stratification. NLP also standardises specialised ophthalmic terminology, facilitating interactions among healthcare providers and patients. With predictions that 85% of customer interactions will be managed without human agents by 2025, NLP-based chatbots hold tremendous potential in triaging symptoms, monitoring treatment adherence, and providing support in areas with limited ophthalmology services.
AI chasm
A core challenge in applying AI is the clinical validation of recently developed concepts and tools. Clinical AI research faces limitations due to retrospective design, leading to biased algorithms “overfitted” to specific data sets. The conventional approach of pitting AI against clinicians may not demonstrate real-world performance, as its realistic application likely involves interaction between clinicians and algorithms. Clinicians, the end users, must grasp AI’s strengths and limitations to foster mutual learning. Envisioning AI’s role in high-risk real-time situations like surgery, where surgeons adapt their approach on the fly, is challenging.
AI regulatory approval is another challenging obstacle. Many algorithms rely on complex and opaque mathematical models, often referred to as “black boxes.” The lack of transparency raises concerns about data mishandling and understanding of the algorithms’ inner workings. Agencies like the FDA/EMA require extensive transparency in scientific methods, but researchers and companies may hesitate to expose proprietary algorithms publicly due to potential financial risks. Further, without a clear understanding of algorithmic processes, AI may struggle to gain patients’ trust and approval. Would it be worse for patients to be misdiagnosed by a human or a machine? What if the algorithm had demonstrated superior performance in research settings? Ultimately, trust and confidence in algorithmic decision-making play a pivotal role.
Overall, more rigorous work is needed to combat the disparity between AI hype and application in healthcare, the so-called “AI chasm.”
The glass cage
The integration of AI into healthcare runs the risk of stripping medicine of its human touch. With advancing algorithms, we may see clinics where machines take the lead, like a macular clinic where an OCT machine decides on intravitreal anti-VEGF injections for AMD without ophthalmologist involvement. Even with fancy AI voice synthesizers like Siri, the empathy and intuition of the doctor-patient relationship diminishes. After all, to these machines, patients are reduced to mere data points where efficiency and cost-effectiveness are the sole measures of success. Further, overreliance on AI-powered automated diagnosis or treatment decisions may stunt the clinicians’ development of critical thinking and decision-making skills, which may, with time, add them to the list of skills lost due to technology.
Biases embedded in the training data can perpetuate inequalities by providing inaccurate or inadequate recommendations for certain groups or populations. With training data predominantly derived in the Western world, algorithms may struggle to effectively generalise to diverse groups. Unchecked, AI can reinforce and amplify existing disparities in healthcare outcomes. Also, the ethical implications of data ownership and privacy protection arise in an era of round-the- clock data collection from gadgets and wearables. Algorithms may exploit this data and stigmatise the chronically ill or those who don’t fit the “healthy lifestyle” mould, potentially leading to unjust financial and health penalties, such as reduced access to insurance.
The rise of AI
We are told AI-powered healthcare is not about replacing ophthalmologists, but rather augmenting their knowledge and expertise. The integration of genomic data, lifestyle factors, and imaging will lead to AI-powered decision support systems that can guide targeted therapies and tailored interventions. Although AI’s current capabilities fall short of the hype, overcoming challenges could unlock its potential in achieving the holy grail of personalised medicine. Yet, the question remains whether the rise of AI leads to the “machinification” of medicine.
Dr Liu is Specialty training year 4 at UCL Institute of Ophthalmology, London, UK. siyin.liu@ucl.ac.uk
Latest Articles
Making Female Leadership More than a Moment
A remarkable global confluence of women in key positions.
ESCRS Talks Technology at AAO
Europe adopts technological advances, US still waiting for lenses and lasers.
Sorting Out Simultaneous Vision IOLs
The ESCRS Eye Journal Club discuss a new landmark paper on IOL classification and the need for harmonisation of terminology for presbyopic IOLs.
Big Advantages to Small-Aperture IOLs
Small-aperture IOLs offer superior image quality with increased range of focus.
Prioritising Self-Care
Benefits of maintaining physical, emotional, and mental health extend beyond the personal sphere.
Valuing Clinical Trial Design
How inclusivity and diversity can enhance scientific accuracy in research.
Knowing Iris Repair: Using Iridodiathermy in Iris Surgery
Prepare for decentred pupils and uneven irides in multiple situations.
Neuroprotectant Treatment for MacTel Type 2
Intravitreal implant releasing ciliary neurotrophic factor found safe and effective in pivotal trials.