Artificial Intelligence
What is the Potential Role of AI in Ophthalmology?
Henahan Prize Finalist Essay
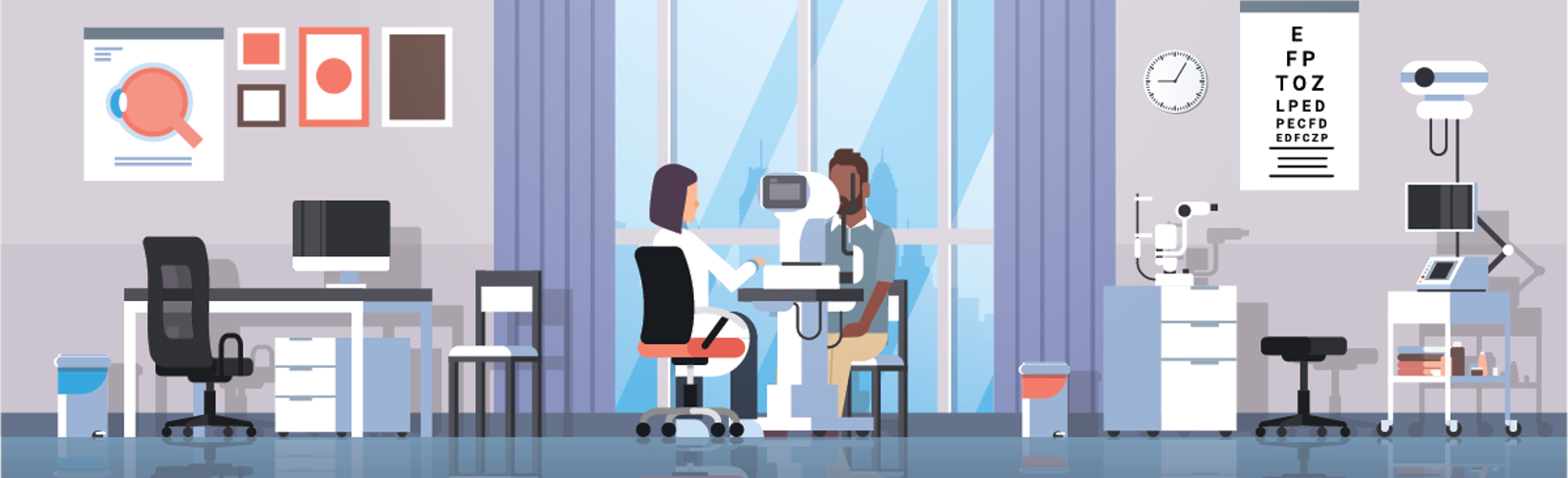
“ Quality of care is of paramount importance, and robust validation is essential to ensure that models are implemented based on true benefit rather than mere hype. “
Dr Thirunavukarasu submitted this essay to the John Henahan Writing Prize essay contest, answering the prompt “What is the potential role for AI in ophthalmology, and what are the negative implications and caveats?”. It was rated in the top 5 of 41 essays submitted by the medical editorial board of EuroTimes.
By Arun James Thirunavukarasu MD
Ophthalmology is at the forefront of medical artificial intelligence (AI) innovation. Through deep learning—where artificial neural networks are trained to process unstructured data such as images and text—many models have been validated for use with fundus photography, optical coherence tomography (OCT), and electronic patient records to augment diagnosis, management planning, and risk stratification.1 Opportunities abound, but risks and limitations must be considered and ameliorated to ensure that AI benefits patients and practitioners.
Opportunities for development
Some AI tools have already undergone validation and acceptance by regulatory authorities such as the US FDA or EU MDR. Progress has been greatest in models for diabetic retinopathy (DR) screening, such as IRIS and IDx-DR.1 For these tools, the aim for development is cost-effective and clinically successful implementation. Economic modelling and systems analysis suggest these models are best used alongside clinicians rather than as a replacement—negative marginal revenue was recorded following autonomous deployment of IDx-DR in the US, but semi-automation (AI triage followed by human assessment) was shown to be cheaper than autonomous deployment or humans working without AI in Singapore.2,3 Benefits of implementation are likely to be greater where human resources are more strained, such as in lower-income countries where access to ophthalmologists is more limited: Cybersight and ARDA have been used to good effect for DR screening of hundreds of thousands of patients in India, Rwanda, and Thailand.4,5 Further work is necessary to identify how other models should be incorporated into ophthalmology services.
However, even where there is better access to care, resources are strained, with waiting lists ballooning around the world in the wake of the COVID pandemic.6 Deployment of AI systems may help address these issues. Large language models (LLMs) exhibit limited ophthalmological knowledge and are unlikely to be deployed as autonomous agents, but they can serve to improve the efficiency of cognitive work by rapidly assimilating information from disparate sources such as clinic notes, correspondence, and investigations.7,8 As multimodal LLMs such as GPT-4 (OpenAI, San Francisco, California, US) and PaLM 2 (Google LLC, Mountain View, California, US) emerge, functionality is set to increase—models can now cope with the rich variety of image-based ophthalmological investigations in addition to large volumes of text. Multilingual models may reduce the requirement for translators and improve communication, improving the equity of service provision with patients of different backgrounds.9 If doctors can delegate time-consuming administrative work to AI assistants, more time can be used to treat patients, improving quality of care and alleviating service pressures.
Risks and limitations
There are a wide range of barriers to AI development in ophthalmology. AI introduces new risks and concerns requiring discussion: cybersecurity (Are AI models using patient data safely, away from adversarial attack?), medicolegal issues (Who takes responsibility for decisions made or contributed to by AI?), and the evolving patient-ophthalmologist relationship as computer systems begin to play a larger role in decision making. Other barriers to implementation are more general. Quality of care is of paramount importance, and robust validation is essential to ensure that models are implemented based on true benefit rather than mere hype. There is room for improvement here, as a 2020 systematic review found just one randomised control trial testing deep learning systems against ophthalmologists, despite an exponentially growing literature base.10 Financial, infrastructural, and human resource demands are significant for AI systems, particularly for initial implementation, and it is necessary to consider whether other interventions may represent a more cost-effective means of improving patient outcomes.
These multifaceted considerations are complicated. To ensure that a fair and optimal outcome is reached for all stakeholders, it is critical that patients and practitioners are consulted. Policymakers must retain neutrality when working with innovators who often have conflicts of interest. Technological limitations must be acknowledged—AI is not a panacea—and evidence-based decisions made regarding precisely where models should (and should not) be deployed. These decisions also depend on ethical considerations, as society must reach a consensus on how AI systems should be incorporated into healthcare systems.
Conclusion
AI systems offer exciting opportunities to automate repetitive cognitive tasks in ophthalmology, such as assimilation, interpretation, and production of data relating to clinical documentation, investigation results, and management planning.1 Automatic DR screening has demonstrated this potential as validated models with regulatory approval are changing clinical practice internationally. Models corresponding to a wide range of other diseases and input modalities are well-established, and implementation is expected to result in significant changes to how eye care is delivered. Policymakers must engage closely with stakeholders to ensure that risks and limitations are mitigated so AI systems work for the greatest possible benefit.
For citation notes, see page 54.
Dr Thirunavukarasu is an Academic Foundation Doctor at the University of Oxford, UK. ajt205@cantab.ac.uk
Latest Articles
Towards a Unified IOL Classification
The new IOL functional classification needs a strong and unified effort from surgeons, societies, and industry.
The 5 Ws of Post-Presbyopic IOL Enhancement
Fine-tuning refractive outcomes to meet patient expectations.
AI Shows Promise for Meibography Grading
Study demonstrates accuracy in detecting abnormalities and subtle changes in meibomian glands.
Are There Differences Between Male and Female Eyes?
TOGA Session panel underlined the need for more studies on gender differences.
Simulating Laser Vision Correction Outcomes
Individualised planning models could reduce ectasia risk and improve outcomes.
Need to Know: Aberrations, Aberrometry, and Aberropia
Understanding the nomenclature and techniques.
When Is It Time to Remove a Phakic IOL?
Close monitoring of endothelial cell loss in phakic IOL patients and timely explantation may avoid surgical complications.
Delivering Uncompromising Cataract Care
Expert panel considers tips and tricks for cataracts and compromised corneas.
Organising for Success
Professional and personal goals drive practice ownership and operational choices.